 |
Validity (Part II)
Last time out, we took a look at the general meaning of "2 key qualities (properties) of 'good measurement.' " These are validity and reliability.
For this "Part II of III," we'll focus specifically on how to demonstrate evidence of validity. As we discussed last time out, since the issue of validity has to do with "unobservables," or constructs that in and of themselves are not directly measurable, we can never "absolutely prove" validity, any more than we can "measure intelligence," "motivation" or any other type of such a construct definitively and without error. The best we can do is make a more-or-less 'convincing case' that based on the evidence, our measurement instrument/procedure is 'valid.'
We'll look at "4 1/2" ways to do so (the "1/2" is kind of "embedded within," or an aspect, of the fourth way -- so it can either "stand alone" in its own right or be a part of # 4, depending on your interpretation and application of it).
The first two of these procedures or methods are quantitative in nature. In fact, they are applications of the correlation coefficient, which we discussed in Intro to Statistics. Therefore, you might wish to review it and/or refer to it from time to time in connection with these first two validity methods.
The third method is primarily qualitative in nature and, in fact, is probably a lot like what you yourselves have already done when you've taught a course for the first time and are looking to develop a "fair" test or evaluation procedure for the students in this course.
Finally, the last one (which contains the "1/2" method) is one that is all-inclusive and very extensive. It is so complex, in fact, that it would be difficult-to-impossible for you, as a sole researcher, to apply this method all by yourself. However, it is entirely reasonable for you to be a part of it -- in essence, adding your "voice" (what you found) to that of colleagues working on understanding different aspects of a given concept, theory or phenomenon.
You may wish to take a few moments to look at the link below, which will give you an overview of the terms used by researchers in discussing validity.
Cornell-Good Discussion about all the "validity" terms.
Let's step through these four methods!
METHODS (PROCEDURES) FOR ASSESSING VALIDITY
- Concurrent (also called Criterion) Validity
This one basically involves the following principle. You develop your own test of "something" (e.g., the desired unobservable construct, such as intelligence) and decide -- if my test performs as well as an existing "gold standard" that's out there, then that is my 'indirect evidence' that my test is measuring what it should (the old "we can't BOTH be wrong ...!" argument!)
In other words, subjects take BOTH 1) YOUR new test (the measurement procedure "being validated") and 2) some existing "gold standard" out there. So, you have 2 scores for every subject.
Then you compare how they "did" on YOUR test with the "gold standard." In other words, for continuous measures, you'd compute a Pearson correlation coefficient between:
a) subjects' scores on YOUR test; and
b) their scores on the "gold standard."
And -- if this correlation is "high" (as per our guidelines on "magnitudes" of r -- generally, better to be as close to 1.00 as possible), that's some (albeit indirect) evidence that YOUR test "performs" the same way (within minor variation or measurement error, of course) as the "gold standard." That's what is meant by "concurrent" -- it stands for "at the same time."
Or, to look at the 2nd popular name, the "gold standard" (existing, extensively standardized/validated measurement instrument) is the "criterion," or "yardstick," by which you are gauging the performance of your own (newer) test/measurement.
Let's look at a specific example:
Let's say that Victoria decides to devise her own "Super-Duper Test of Intelligence." She decides to check its performance (is it classifying subjects 'the same way,' again within minor, acceptable variation and measurement error) as a more established, extensively validated "gold standard?" In this way, she will determine the "concurrent" or "criterion validity" of the Super-Duper Test of Intelligence.
So:
- She selects the Wechsler I.Q. test as her gold standard;
- She randomly draws a sample from a well-defined population of interest; say, 100 subjects;
- She gives 50 of them the Wechsler test first and then Victoria's test; and the other 50 get Victoria's test first and then the Wechsler (P.S. Do you see why this is necessary? To prevent a possible "order effect," or spillover/contaminant if they all took the SAME test FIRST);
- She takes the resulting 2 scores for each subject and then calculates a Pearson correlation coefficient (if hers is scaled continuously; let's assume it is).
- If the resulting Pearson r is "high," or "acceptable" -- let's say it's +0.89, which would be great -- this is Victoria's evidence that her Super-Duper I.Q. Test has concurrent or criterion validity as compared to the Wechsler.
P.S. Please note that, with regard to a "good" value of the correlation coefficient, not only do we need a strong magnitude (size) of r -- but the direction, or sign, had better be positive!
For instance, suppose we got a -1.00 value of r between Victoria's test and the Wechsler. Well, that would be disastrous for poor Victoria! It would mean her test is classifying Wechsler high I.Q.'s as bottom-level I.Q.'s and vice versa! In other words, exactly the opposite of Wechsler!
Please keep in mind, again, that the "strength of conviction" of this argument hinges on how much "faith" there is in the "gold standard" to begin with! Not all fields or areas have such well-established "gold standards." And -- there is certainly the remote chance that the 2 tests could BOTH be "wrong" with respect to the underlying construct!
But for areas where such "gold standards" do exist, this has been found to be a convenient way to gauge the performance of the new test or measurement vis-a-vis the old.
One final, rather 'common-sense, mince-no-words' comment -- and it may have occurred to some of you -- you might wonder, well, if there IS a "gold standard" out there, then why should Victoria even be going to the trouble of devising a new instrument to begin with?!
This is a good question and the answer comes down to simple economics or efficiencies! If Victoria's test represents some sort of time/cost "savings" (e.g., shorter form; easier to administer or take) AND we can show it performs " just about as well as" the "gold standard" (via our calculated r, or numerical evidence of concurrent/criterion validity), then by all means, it would make more sense, time- and money-wise to use Victoria's test!
- Predictive Validity
We've also seen this one in our Intro to Statistics Module # 1! It says, "If my measurement does a 'good enough job' of predicting my desired outcome, then I will consider it to have 'high', 'good', or 'acceptable' predictive validity."
Another way of saying that might be, "If it 'works well' (as a predictor), then it will be assumed to be 'valid' (as a predictor)."
Let's look at a graphical example of this.
As with our other "predictive" type examples in our research and statistics modules, we need a situation where the measurement (predictor) precedes the desired (criterion) target outcome variable. As has been shown to be the case through repeated correlations, the typical college entrance tests have a pretty good (although not perfect, as we know! Too many other possible contaminating variables) "track record" with regard to predicting first-year college GPA (as the observable indicator of "potential for successful college-level work"). Thus, we would say that it has "good predictive validity."
This, then, is the idea in predictive validity:
- Identify your predictor and criterion (target outcome) measurements and make sure the first precedes the second in time;
- Correlate the resulting measurements on both variables.
Again, a "high" value (magnitude) of r would imply "good" or "acceptable" predictive validity. And in this case, I suppose the sign could go in the opposite direction, depending on the underlying properties of the two measurements or variables being correlated. For instance, I could picture a strong NEGATIVE predictive validity correlation coefficient between:
- "current debt level owed or carried" (predictor); &
- "credit-worthiness to carry more debt" (criterion).
That is: the more you owe now, the less debt you can probably take on. This is undoubtedly a factor in credit card companies', banks', and other lenders' decision to extend credit.
Also, one final comment here about predictive validity. The punch line really is, "If it works, 'use it' and assume it's valid." But there is no guarantee that the "good predictor" is "strongly THEORETICALLY connected" to the "criterion" (thing being predicted).
For instance (dumb example but it might serve to make the extreme point!): if it happened to be the case that "eye color" or "shoe size" highly correlated with first-year college G.P.A., then we'd conclude, via THIS method, that it has "good predictive validity!" Yet, even though it "works," eye color or shoe size is really not "connected in any common-sense way" to G.P.A.!
Some researchers might not find that too palatable if they're wanting to "build theory" or "really conceptually understand" a construct. (And that's where our last "2 1/2" methods will come into play! They will rely much more on "common sense!")
- Content (also called "Face") Validity
This is a qualitative "judgment call" rather than a correlation or other quantifiable indicator of validity! It means, "I'll assume my test (survey, interview protocol, other measurement) is 'valid on the face of it.' My evidence for such a judgment call will be feedback from 'expert judges,'as well as my own research into relevant books, articles, etc.!"
Let's say you're a brand new first year algebra teacher. It's time to devise your midterm test for your algebra class.
You might start by looking through a number of books, curriculum guides, teachers' manuals, etc., to see "what's a 'fair and complete' test" of the concepts (e.g., "skill in polynomial equations") that you wish to assess.
Also, you very well might huddle with other algebra teachers, at your school/district and maybe solicit the help of friends. You might ask them to review and "critique" a draft of your test and then modify it to reflect "what's too advanced? too basic? what about order and wording of items? time allotted for students to complete them? etc., etc.?"
After consulting all such 'sources of established evidence' as to what a 'good' test of these midterm algebra concepts should contain, you'd write and probably revise your own test accordingly. You might also need and want to bounce a revised draft off your teacher-colleagues to see if "you're now in the right ballpark" with regard to some of their suggestions.
At that point, you'd be making the qualitative judgment call that your instrument as "high," "good" or "acceptable content, or face, validity."
The following is a general schematic of this process:
This particular procedure for pilot testing, or demonstrating the validity of a measurement instrument, is probably the most popular one applied for doctoral dissertations. It doesn't require the often-massive numbers of pilot-test subjects that may be needed by the more quantitatively oriented procedures.
And, although some researchers object to the "looseness" and "qualitative nature" of the above, it actually turns out to be more revealing than the numerically based procedures. For instance, judges and/or existing sources will give you solid "contextual" type information regarding "what's A-OK and what can be rewritten to be better." A correlation of 0.89, on the other hand, is certainly outstanding in terms of a "level" of demonstrated validity. But it doesn't tell you "what could have been done to make it even higher."
FINAL TIP: I've written a paper illustrating how to conduct a pilot test of instrumentation using expert judges in order to establish qualitative evidence of content/face validity. You might like to get a copy of this paper: the models and procedures might be useful to you at prospectus and dissertation stage when you wish to validate your own instrumentation!
This paper is entitled, When 'Do It Yourself' Does It Best: The Power of Teacher-Made Surveys & Tests. It is currently on reserve in Cline Library under EDR 725 - Qualitative Research and Analysis Procedures - Dereshiwsky. Might be worth going over, finding and Xeroxing it. Or, you can access it from your local library: it has been published in the ERIC database, as well.
- Construct Validity
Open and read this one page introduction to Construct Validity.
Construct Validity
This is the one that's a lengthy process - most likely, not achiveable by a single researcher in one time and place.
In construct validity, we are trying to develop a theory about a particular unobservable construct. That is: we wish to understand: what makes it what it is? and under what particular circumstances (persons, groups, situations, settings, other variables) does it vary or differ?
So many of these are still in progress! What is intelligence? motivation? satisfaction? and so on and so forth!
Now ... your study might contribute a key missing link to the overall "puzzle" of understanding a given construct! If so, you "become part" of the overall construct validation of that construct!
Ellen might start by uncovering, through a well-designed and executed study, that satisfaction varies by job level (clerical vs. administrative). Then Billie builds on that and also finds a key gender difference within each type of job level. Finally, Susan discovers that age and experience are significant correlates as well!
Do you see how each of the preceding, seemingly "stand-alone" research studies on satisfaction, we can also "patchwork-quilt" them together to gain a more revealing overall picture with regard to "what is satisfaction?" and, in particular, under what circumstances, or conditions, does it vary?
This, then, would be the ongoing process of emerging a theoretical explanation of a construct: e.g., painstakingly continuing to validate or understand that construct by continual, successive, refined research by different persons in different places at different times!
And now for the "1/2" embedded within this 4th overall method of validation!
4 1/2. Discriminant Validity
That would be Billie's example of finding a gender difference! Or Susan's regarding type of job!
That is -- this sounds backwards in thinking, I know; but please bear with me! -- if we can use a "measurement" or "score" to "separate respondents into groups," that would imply that the measurement procedure has "good discriminant validity!"
In other words, knowledge of the score (its level) helps us "discriminate" or separate the subjects into groups according to that score!
This would mean that, on average, we would expect "strong" or "large" between/among-group differences on that score.
Extreme example, but it makes the point:
If the "score" is, say, "attitude towards abortion" and the "groups" are "liberal" vs. "conservative," we certainly would expect "good discriminating ability" or "separation" (e.g., "discriminant validity") by this score! We'd be able to pretty well classify the "negative attiude" scores as the "conservatives" and the "positive attitude" scores as the "liberals!"
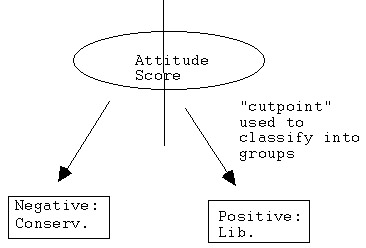
The challenge, of course, is to determine that "clean cutpoint," or score for which we can say:
- if subject A scores at or below this value, classify him/her as "conservative;" or;
- if subject A scores above this value, classify him/her as "liberal."
In fact, there is such a statistical procedure -- and it is known as "discriminant analysis!"
In looking back on it, not only can discriminant validity be a part of overall construct validation (e.g., Billie's 'discovery' of the 'gender difference' with regard to satisfaction level) - but via this classification procedure (into groups based on scores) we can also consider discriminant validity to be a special case of # 2, predictive validity (since we are predicting group membership of a subject based on his/her score).
That's "all she wrote" (meaning STATCAT, of course!) for validity!
We'll wrap up the glorious cyberspace research adventure by looking at analogous methods for assessing reliability in upcoming lesson in Part III of Validity! (And aren't we getting "pretty darned high-powered ... ?!")
Once you have completed this assignment, you should:
Go on to Assignment 1: More Mission "Possible"
or
Go back to Validity and Reliability - Part II
E-mail M. Dereshiwsky
at statcatmd@aol.com
Call M. Dereshiwsky
at (520) 523-1892

Copyright 1998
Northern Arizona University
ALL RIGHTS RESERVED
|